

#Gplab toolbox series
ĭe-Falco, I., Della-Cioppa, A., Tarantino, E.: A genetic programming system for time series prediction and its application to el niño forecast. In: Proceedings of the 14th IEEE Signal Processing Society Workshop Machine Learning for Signal Processing, pp. Guo, H., Jack, L.B., Nandi, A.K.: Automated feature extraction using genetic programming for bearing condition monitoring. ĭermofal, D.: Time-series cross-sectional and panel data models. Poli, R., Langdon, W., McPhee, N.: A Field Guide to Genetic Programming. Experiments are conducted on different benchmark problems to highlight the advantages of this new approach. In this work, we perform a comparative analysis of vectorial GP (VE-GP) against standard GP (ST-GP).

This new representation allows aggregate functions in the primitive GP set, included with the purpose of describing the behaviour of vectorial variables. To maintain the source of knowledge supplied by ordered sequences as time series, we propose a new approach to GP that keeps instances of the same observation together in a vector, introducing vectorial variables as terminals. However, representing data in this form may imply a loss of information: for instance, the algorithm may not be able to recognize observations belonging to the same subject and their recording order. To manage this problem with GP data needs a panel representation where each observation corresponds to a collection on a subject at a precise time instant. A common situation consists in the prediction of a target time series based on scalar features and other time series variables collected from multiple subjects. Amsterdam: Elsevier Science.Among the various typologies of problems to which Genetic Programming (GP) has been applied since its origins, symbolic regression is one of the most popular. The economics of information systems and software. Data mining: Practical machine learning tools and techniques. IEEE Transactions on Reliability, 62(2), 434–443.
#Gplab toolbox software
Using class imbalance learning for software defect prediction. New York: Vieweg and Teubner Verlag Springer. A learning system based on genetic adaptive algorithms. An empirical study of some software fault prediction techniques for the number of faults prediction. Zero-inflated poisson regression, with an application to defects in manufacturing. In Proceedings of the 2007 conference on emerging artificial intelligence applications in computer engineering: Real word AI systems with applications in e health, HCI, Information Retrieval and Pervasive Technologies, The Netherlands, pp.
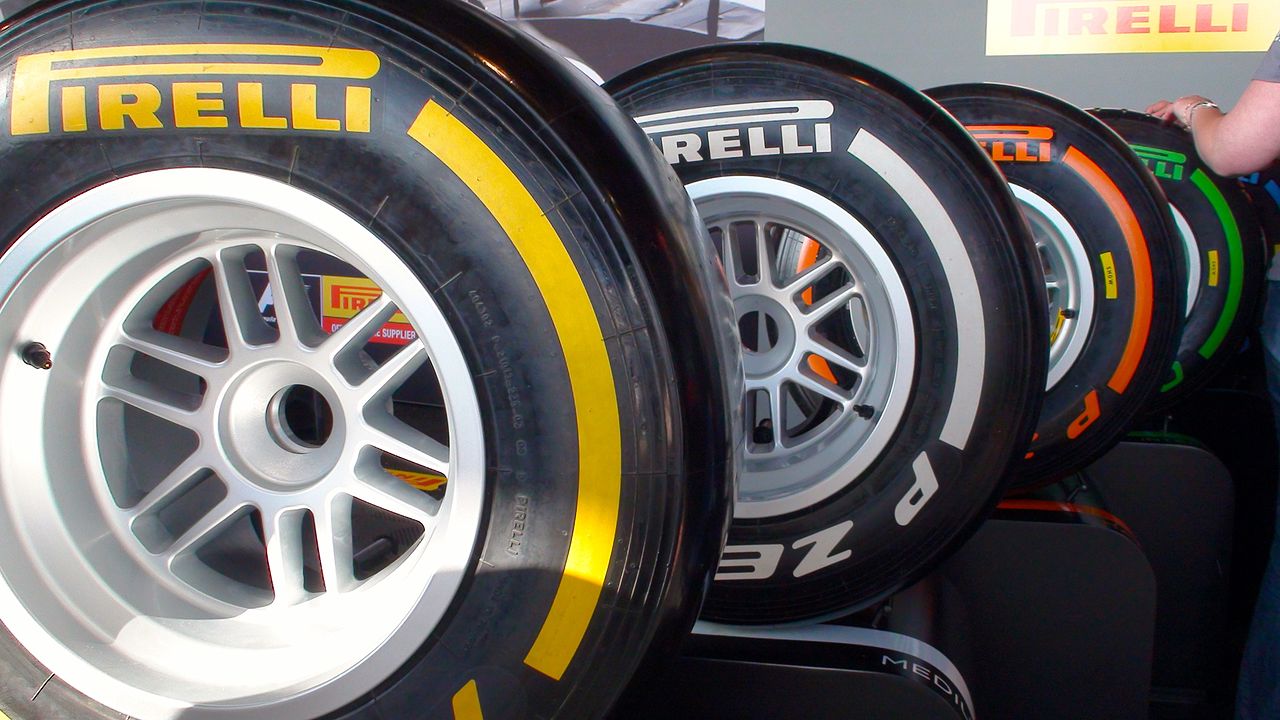
Supervised machine learning: a review of classification techniques. California: Jet Propulsion Laboratory California Institute of Technology and Arizona State University. Boston: Addison-Wesley Longman Publishing Co.Inc. Genetic algorithms in search optimization and machine learning (1st ed.). In 7th IEEE Working Conference on Mining Software Repositories (MSR), pp. An extensive comparison of bug prediction approaches. London: Routledge.ĭ’Ambros, M., Lanza, M., & Robbes, R.

Applied multiple regression and correlation analysis for the behavioral sciences (3rd ed.). Cambridge: Cambridge University Press.Ĭohen, J., Cohen, P., West, S.
